Matching the right buyers, to the right products, at the right time
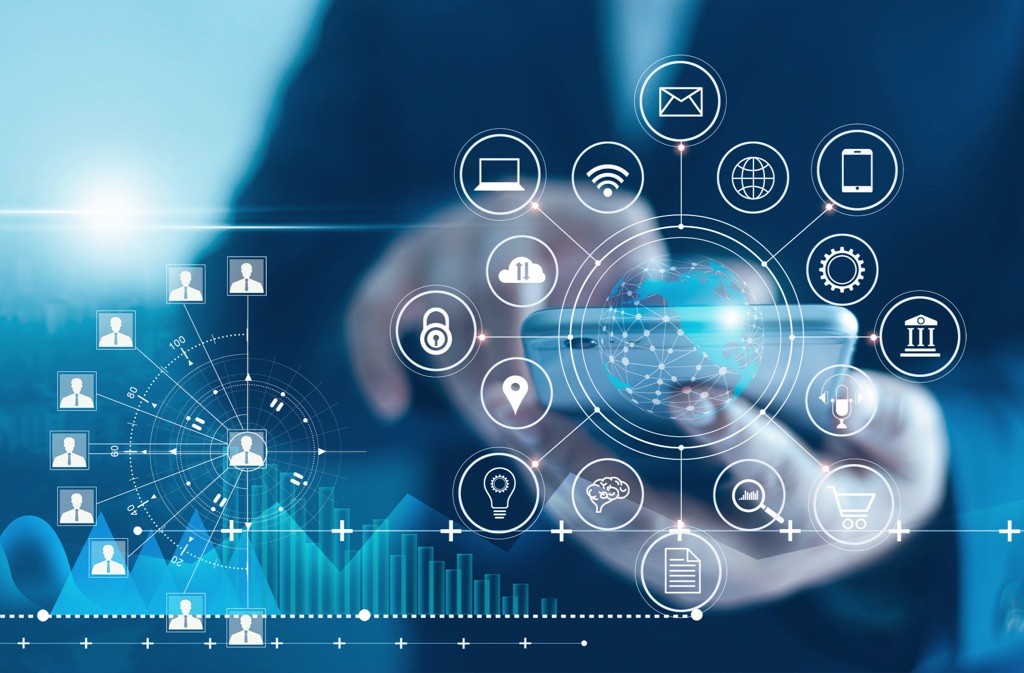
You don’t need to be an auction theorist to know that getting the right people in front of the right inventory, at the right time, is the key to trading goods with the people that value them the most.
Similarly, auction theory explicitly shows how price is optimised by maximising the number of bidders participating in the price discovery mechanism. If participation is maximised, goods are more likely to be traded with the bidders that value them most. While these are easy concepts to grasp, they are harder to implement systematically within an online marketplace.
However, buyer-to-seller matching magnetises buyers of goods to sellers with the most attractive inventory based on given criteria. Likewise, sellers receive signals based on the most appropriate buyers for their goods. The aim is to find the best mix of market participants to invite to an online ‘trading room’, to achieve the best possible prices through the sale of goods to those that value them the most.
This problem is acute when there are hundreds of buyers largely unknown to a community of hundreds of sellers, each of which having hundreds of items of inventory for sale.
This situation often leads to a marketplace with a substantial number of listings available for sale at any one point in time, requiring considerable time and effort to browse and surface the listings of greatest interest. Ultimately, this leads to a marketplace that is ‘congested’ with potential trading opportunities, which leads to fewer bids, sub-optimal pricing, and weary traders.
To mitigate this, NovaFori applies machine learning to historical trade data to build intelligent algorithms that match the most appropriate buyers to sellers.
Specifically, our matching algorithms can work alongside any ‘hard coded’ business logic that needs to be present. For example, buyers and sellers can be filtered for eligibility by jurisdiction, credit worthiness, and many other metrics. The idea is that only those counterparties who are actually able to trade need to be notified of an opportunity.
We then produce a list of eligible buyers that are most likely to place a bid for goods available for sale. Likewise, we produce a list of sellers that are most likely to be able to fulfill the order for goods desired by buyers. These lists can be used to automatically invite market participants to engage in a trade, or to actively recommend inventory within a web application (i.e., as a recommendation engine).
Thus, the number of buyers and sellers invited to participate in a trade can be automatically chosen so there is no expected increase in final price from notifying additional participants. The idea is to strike a balance between achieving optimal price discovery for a single trade listing, and ‘spamming’ all market participants, saturating their attention and congesting the marketplace at the detriment of all other trade listings. This strategy is based on classic results from auction theory as well as our experience spanning many marketplaces. As a result, this type of thinking is baked into our matching algorithms.
Getting the right people in front of the right inventory, at the right time, is the key to trading your goods with the people that value them the most, simultaneously optimising the price discovery process. As marketplaces move online, the number of trading opportunities and signals are multiplying at an exponential rate. Thus, this seemingly simple process is prone to the ‘inefficiencies of scale’.
NovaFori’s intelligent marketplace technology provides the solution by automating the process of matching buyer to sellers.
To learn more about matching buyers and sellers in your industry get in touch.
Sign up
Subscribe to receive our blogs, white papers and media articles.